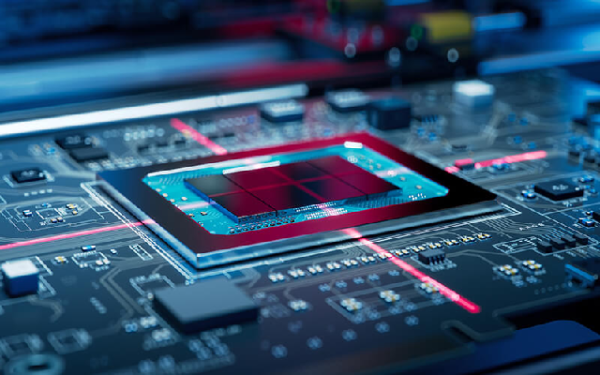
Artificial intelligence (AI) is revolutionizing how we interact with technology. From language translation to autonomous vehicles, it is rapidly becoming an integral part of our daily lives.
AI refers to the development of software and hardware for machines to perform tasks such as machine learning, deep learning, data analysis and real-time decision-making that previously required human intelligence. For the most part, generative AI such as ChatGPT, which focuses on creating content and comes in a dialogue format, can be found in data centers. It has been used to write essays, paint pictures and compose symphonies. But can it be used to design circuits?
The application of AI techniques in EDA is still in the early adopter phase, with design engineers exploring use cases and using AI to simplify complex problems. AI can be particularly valuable in model development and validation for simulation, where it assists in processing large volumes of data.
The Promise of Generative AI
By leveraging machine learning algorithms, generative AI can automate the design process, enabling rapid prototyping and investigation of the design space. In chip design, generative AI can automate layout and floor planning; optimize performance, power and area (PPA) and ensure adherence to design rules. It can identify optimal solutions that traditional methods might overlook and streamline the verification process, reducing errors and improving design quality.
Emerging AI features in electronic design automation tools are freeing engineers from tedious tasks such as layout, optimization and simulation and allowing them to apply their ingenuity toward solving more difficult problems.
If you have doubts about what AI can do regarding chip design, the following recent news items, not necessarily presented in the exact order of arrival, will erase them.
Siemens Digital Industries Software, for example, has introduced Solido Design Environment software, an AI-powered, cloud-ready integrated circuit (IC) design and verification solution that can help design teams meet increasingly aggressive power, performance, yield and reliability requirements while helping to speed time to market.
The Solido Design Environment uses AI to perform what the company calls “number crunching on steroids” that reduces the number of simulations needed to verify a design and optimize the chip’s power, performance, layout and yield. Solido can run simulations, view waveforms and perform regression analysis on analog and digital custom IC designs.
Solido takes the same component models and input data that an engineer would enter into a typical simulation tool and from that data build its own custom machine learning model to determine the optimal sampling and testing conditions to evaluate the robustness of the chip design. Siemens says that this could reduce future verification times by one to two orders of magnitude.
Turning to system-level design, SnapMagic’s Copilot brings the power of generative AI to electrical engineers. By specifying a design using natural language, much like typing a query into ChatGPT, the tool can optimize the BOM and even determine whether a component will be difficult to obtain due to supply chain issues.
According to SnapMagic, an engineer can add a microcontroller to a circuit and the AI tool will automatically place the recommended decoupling capacitors. It can also suggest manufacturer-recommended reference designs.
With the introduction of Synopsys.ai Copilot, Synopsys is harnessing the power of generative AI to provide design teams with new levels of productivity. This capability is the result of a strategic collaboration with Microsoft to integrate Az Azure OpenAI Service, bringing the power of generative AI into the design process.
Initially, Synopsys.ai Copilot will serve as a knowledge query system, pulling from Synopsys resources such as product user manuals, application notes, videos and any documentation found on the Synopsys SolvNetPlus support community. Queries can range from simple questions (“Which option should I use?”) to more complex and specific questions that might entail follow-up questions by Copilot.
Cadence, has announced the industry’s first large language model (LLM) for chip design (a proof of concept project named ChipGPT) and the OrCAD X Platform for PCB design.
The Cadence JedAI LLM focuses on the design-cleaning process. Engineers can use the LLM chatbot to interrogate the design, validate the design versus specification, explore and rectify issues, prompt analysis tasks and receive explanations in their natural language.
Defect Detection Using AI
In the past, passive component manufacturers mostly conducted appearance defect detection using six-sided inspection machines based on automated optical inspection (AOI) technology, which compares optical images of items with prescribed rules to identify defects. Unfortunately, such a rule-based inspection method can be prone to missing defects.
Since AI can learn the characteristics of defects from large amounts of historical data and identify defects through those characteristics, AI is superior at defect detection and can detect more defect types than AOI.
So, to overcome some of the limitations of AOI technology with artificial intelligence and improve production yields, companies such as Spingence in partnership with Advantech have launched the AINavi defect detection software to maximize defect detection performance.
AINavi runs on Advantech’s MIC-770 system with a graphics card, or it can run on the MIC-730 AI inference system. AINavi can inspect thousands of parts in just one minute, meeting the high-speed inspection needs of production lines.
In conclusion, the overall market for generative AI is booming. With the implementation of generative AI, chip, system and product design are poised for unprecedented advancements and innovation. AI promises to effectively shorten design cycles and, in some cases, eliminate the need for custom, specialized components.
Source: Murray Slovick (TTI)